Artificial Intelligence (AI) is no longer a futuristic concept; it is a present-day reality shaping businesses across the globe. According to Gartner, approximately 80% of enterprises will have used generative AI (GenAI) APIs or models by 2026. This trend underscores a growing recognition of AI’s potential to drive value for organisations, further fuelling its demand and adoption.
Indeed, the latest SME IT Trends Report from ManageEngine reveals that 87% of surveyed organizations plan to use AI, with only 13% having no plans for AI initiatives. The enthusiasm for AI is evident, with 61% of respondents expecting to implement AI initiatives within the next year, 76% agreeing that their organisation should invest in AI, and 63% have already developed an AI policy.
However, integrating AI is not without its challenges. Large language models (LLMs) delve into complex subjects but are limited by the data they can access, often leading to superficial or incorrect outputs. Hence, accuracy and context are critical, as the results generated by AI drive business and security decisions.
Martin Hartley, Group CCO of Emagine, emphasises the importance of data quality in AI success. “If you have all the right metrics to build the model but not the right tools, there will undoubtedly be flaws in the system. Starting with poor quality data that hasn’t been structured or cleaned will lead to an AI system that crumbles later down the line. AI needs structured data to perform any task it is set up to do, so it needs to be the number one priority for teams.”
Photo Credit: James Robinson
Echoing this sentiment, Dominic Wellington, Enterprise Architect at SnapLogic, highlights the perils of poor data management, citing the ‘Savey Meal-Bot’ incident in New Zealand, where an AI chatbot recommended dangerous recipes due to being trained on bad data. Ensuring data accuracy and security is paramount to prevent such failures and to build user confidence in AI tools.
AI for your business
Max Belov, CTO at Coherent Solutions, provides practical examples of AI’s successful implementation. Companies like Amazon and Netflix use AI for product recommendations, while logistics companies like UPS leverage AI for supply chain management. AI’s applications extend to customer service, with AI chatbots enhancing customer experiences and cybersecurity firms integrating AI into their security testing products.
However, Belov also identifies common challenges in AI implementation: ensuring data quality and availability, finding skilled personnel, integrating AI with existing systems, navigating ethical and regulatory concerns, and managing costs and uncertain ROI. Overcoming these obstacles requires robust data management practices, upskilling staff, and adopting phased implementation approaches with clear business value metrics.
Belov advocates for cross-functional teams to ensure AI project success. These teams, or ‘pods,’ should include product management, architecture and design experts, diverse engineering resources, data and analytics specialists, quality engineers, and security experts. Involving ethicists and legal advisors is also crucial to ensure adherence to ethical standards and regulatory requirements.
Alexandra Mousavizadeh, CEO and Co-founder of Evident Insights, outlines how businesses can measure AI project success. “Establishing organisation-wide measurement frameworks is essential, with metrics falling into five categories: income uplift, efficiency gains, risk reduction, customer satisfaction, and staff satisfaction. Continuously measuring AI projects against a common framework ensures accurate outcome assessments.”
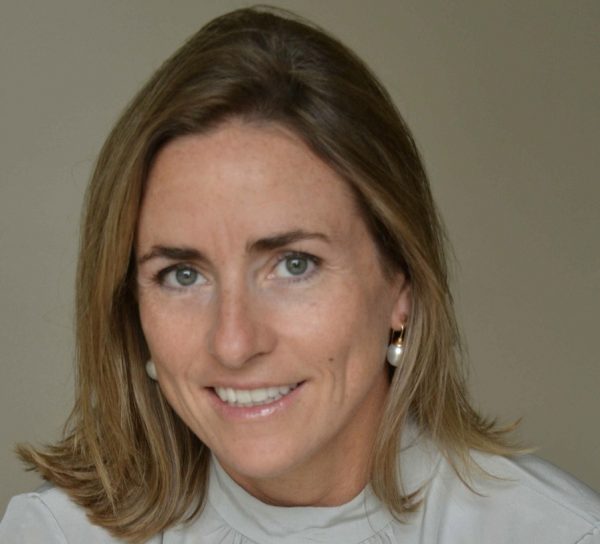
Tristan Shortland, Chief Innovation Officer at Infinity Group, discusses emerging AI trends that companies should be aware of. “The growing investment from businesses like Microsoft, OpenAI, and Google, among others, in AI is here to stay. We are already seeing the power of Generative AI and LLMs filtering through into the workplace. This is only going to gather pace and extend more globally into day-to-day working lives as more organisations adopt the technology. We are also seeing the rise of more roles focused on AI being created in organisations. Many of our own clients are now starting to prioritise this within their talent acquisition plans to extract long-term value from their AI investments.”
Shortland highlights trends such as the democratisation of AI with smaller LLMs, the prioritisation of responsible AI to ensure fairness and transparency, and the rise of AI on devices, enabling advancements in edge computing and IoT. Additionally, the development of multimodal AI, which can process multiple data types, will further enhance AI’s capabilities.
The data question
AI thrives on data. The quality, quantity, and relevance of data are crucial determinants of the success of any AI initiative. Organisations must invest in robust data management practices, ensuring data is clean, accurate, and accessible. This involves the technological infrastructure for data collection and storage and the governance frameworks to maintain data integrity and compliance with regulations. With a solid data foundation, AI models can be trained more effectively, leading to more accurate and reliable outcomes.
AI adoption is as much about cultural transformation as it is about technology. To fully realise AI’s benefits, organisations must foster a culture of innovation and continuous learning. This means encouraging experimentation, tolerating failures as part of the learning process, and promoting cross-functional collaboration. Employees at all levels should have the skills and knowledge to work alongside AI technologies. Investing in training and development programs can demystify AI, making it a tool for empowerment rather than a source of apprehension.
Ethical considerations are paramount in AI deployment. The potential for bias, privacy concerns, and unintended consequences must be proactively addressed. Organisations should establish clear ethical guidelines for AI use, ensuring transparency, fairness, and accountability. This involves regular audits of AI systems to detect and mitigate biases and implement measures to protect user data. Ethical AI practices build trust with stakeholders and mitigate risks associated with regulatory non-compliance and reputational damage.
Extracting value from AI investments requires a strategic, well-rounded approach. It’s not merely about adopting the latest technologies but about embedding AI into the very fabric of the organisation. By understanding business needs, building a strong data foundation, fostering an innovative culture, ensuring ethical practices, integrating AI into processes, measuring value, scaling solutions, collaborating with partners, and preparing for future trends, organisations can unlock the full potential of AI. This comprehensive approach will enable businesses to achieve immediate benefits and sustain long-term competitive advantage in an increasingly AI-driven world.
Leon Gauhman, Co-founder and Chief Strategy Officer at digital product consultancy Elsewhen.
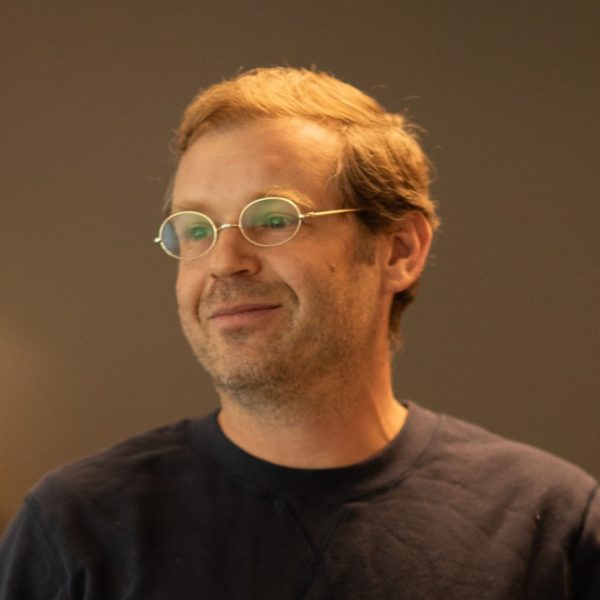
What are the key factors a business should consider when deciding to invest in AI technologies?
“There’s a lot of confusion about what investing in AI technologies means. It’s fine to allow employees to dabble with generative AI tools to see how they can improve productivity. But doing so does not mean companies are investing in AI.
“Building a tailored AI solution represents a significant investment for companies across the board – time, patience, and money are all at a premium. So, business leaders must begin with a clear vision of what they want to achieve with such a move. Key factors to assess include:
- How will this innovation impact company profit and revenue? What’s the point of building AI muscle into our architecture? Can this tool unlock business challenges – or radically transform how we do things? Companies need to be able to articulate the business case for AI technology based on the clear benefit it will bring to their balance sheet.
- Can we ensure that our business won’t be put at risk by our intended AI integration? To use the medical adage, first do no harm.
- Do we have the time and resources needed to utilise AI technologies in a robust way? And do we have access to the right talent – people who can rely on their in-depth understanding of AI capabilities to create relevant use cases for our business?
Can you share some examples of how companies have successfully extracted value from their AI investments?
“One of the most powerful ways AI and LLM tools can be applied is in terms of productivity and alleviating the digital workload – by freeing up time for employees to focus on more satisfying, innovative and value-add activities. For example, we recently reduced the workload of a major media and advertising client, by offloading key processes to AI.
“Elsewhere companies are successfully extracting value from AI agents that support customer service employees. Klarna’s AI assistant recently handled the equivalent work of 700 full-time customer service agents in one month alone, prompting a 25% drop in repeat inquiries and driving an estimated $40 million USD in profit improvement this year.
“Similarly, the AI @ Morgan Stanley Assistant helps support staff across the bank digest over 100,000 research reports. At the same time, its latest AI assistant tool, Debrief, is predicted to save thousands of hours of labour for the company’s 15,000 wealth advisors by this summer by handling menial tasks such as note-taking, meeting logs and automated email drafts.
“AI is also linked to longer-term revenue, helping organisations fundamentally rethink their business models and how they create value. This is seen, for example, in global publishers licensing their content to OpenAI or Elsevier creating a generative AI-powered database based on their proprietary academic content.”
What strategies do you recommend for aligning AI initiatives with overall business goals to maximise return on investment?
“AI has a three-fold business impact: cost savings, redesigning work, and, in the longer term, crafting new revenue streams. This means that AI initiatives should be discussed at the board level, and boards should ensure that technologists—people who deeply understand AI and other digital technologies—have a voice at the top table.
“Business leaders should define clear KPIs for their AI projects, aligning them with strategic objectives and providing measurable targets. These will serve as the benchmarks for evaluating the success and impact of specific implementations.
“However, the hype aside, AI is just another technology. So, businesses can leverage the same trusted strategies that apply to working with any other emerging technology including design thinking and lean, agile methodologies. These methodologies provide reliable tools and frameworks that allow stakeholders to test ideas, collaborate and see tangible outcomes early on. The aim is to learn fast, and release continual value. Doing so ensures that AI and broader business goals are aligned.”
What common challenges do businesses face when trying to implement AI, and how can these be overcome?
“One key problem is that, because of all the hype around AI, businesses fail to think about it as just another new technology. Companies have successfully negotiated new technologies before, including the web and the rise of cloud computing, and AI is simply the next stage of technological change. Like any other new technology, there are several challenges at play with AI—from cybersecurity to managing ethical risks.
“Businesses’ immediate response is often to be scared of AI’s apparent dangers. Whereas what companies really need is a cool-headed assessment of the huge benefits that this new tech wave could bring. The real risk lies in doing nothing and being left behind. Another challenge is to develop long-term thinking. Change via AI is a major commitment, and expecting a return in one quarter alone is unrealistic.”
How important is it to have a cross-functional team involved in AI projects, and who should be included in such teams?
“AI technology is incredibly complex, and it takes many great minds to work together to create something worthwhile. To navigate this challenge strategically, siloes are out. Instead, companies need to get lots of different people with diverse skills on board.
“With a cross-functional team, everyone will bring their perspective and abilities to solve the problem. Engineers can have input on design, and vice versa. Product and business experts also need to be in the mix, working collaboratively to deliver better outcomes under the banner of a common goal.
“The C-suite has a vital role to play here, too. Business leaders must create the support that allows their teams to prove value within a realistic timeline. It’s not possible to tackle AI as a side project on top of BAU work. Rather, teams need ample time and budget to implement solutions successfully and responsibly.”
What best practices can you suggest for ensuring ethical use and avoiding biases in AI applications?
“The obvious answer is always to have a human in the loop to monitor and account for emerging risks. So often these days, we hear talk of AI replacing humans. In reality, the two will always work best in tandem. That’s especially true in relation to more thorny AI issues — such as systems that inadvertently scale biases. In cases with a particular risk of ethical error, it’s also an idea to introduce multiple systems designed to cross-check one another.
“In addition, business leaders must encourage a constructive and proactive dialogue around AI risk. But again, it’s important not to overstate potential dangers. AI is just another chapter in digital transformation, and decades of software development mean we are well-placed to navigate any emerging ethical, regulatory, and security issues that may crop up en route.”
How can businesses keep up with the rapid pace of AI advancements to ensure their investments remain relevant and valuable?
“AI is advancing at breakneck speed, led by a series of groundbreaking moves and countermoves from the likes of OpenAI, Google, and Anthropic. As a result, the boundaries of our digital environments are constantly shifting.
“To keep up, businesses must invest in the people, resources, and timelines needed to continually test new tech. Ideally, businesses will have tech teams capable of quickly trialling emerging tools and either discarding or developing them, depending on whether they are relevant to business growth. This capability requires significant investment, supported by boards that are prepared to engage with AI in-depth. Most organisations aren’t yet at this point.”
Looking forward, what emerging AI trends should companies be aware of to continue extracting maximum value from their AI investments?
“Multimodal AI — that is, the integration of several specialised models that work in unison—is set to be big. We can see examples already with Google’s Gemini, a model that operates across code, audio, image, and video inputs simultaneously. Multimodal architecture represents a step towards AI that can manage more conceptual, nuanced, and complex reasoning. It’s more suited to capturing a wide human corpus of knowledge and experience.
“We’re also seeing a move beyond chat interfaces to deeper, more creative integrations and state-of-the-art UX. GPT4.o and Project Astra show just how personalised and proactive conversational interfaces can be. They’re able to communicate in a more human, contextually relevant way than previously possible: Again via a combination of vision, voice and text. This emphasis on user experience will soon be enhanced by learnings from millions of active users (for example, on ChatGPT) – insights that can be used to shape increasingly imaginative and intelligent interfaces.
“What this means is that, increasingly, we will see the heavy lifting of interacting with AI — specifically, the complex task of prompting — move behind the user interface. This shift will allow users to focus on their work, rather than on how to communicate with the AI.
“Finally, we’re seeing a race emerge in the commoditisation of foundation models – driven by the proliferation of Open-Source solutions. From now on, we’ll see a constant stream of new and specialised AI models that draw on Open-source and synthetic data. Companies operating in this world will have more choices and challenges than ever.”